Your AI Data Pipeline
We provide the tools and know-how to help you make the most of your AI-based solutions. Good models require good training data. Together with our partners, dSPACE offers all the tools and services required for your data pipeline. Gather new data with AUTERA and RTMaps, annotate the data with the UAI Annotator, and manage the data in IVS. This process goes hand in hand with our tools for creating arbitrary traffic situations, for example, by using Scenario Generation from Measurement, and finally for generating additional training data from these scenarios using the dSPACE solution for sensor-realistic simulation.
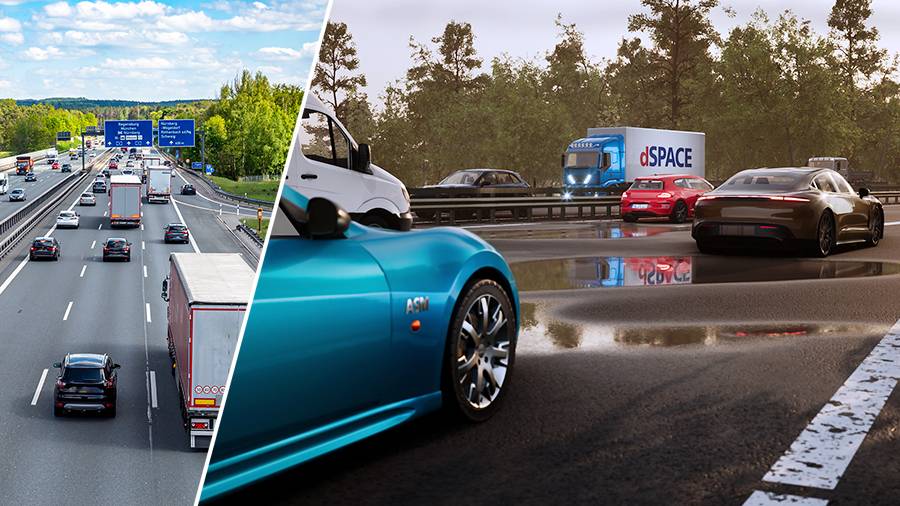
How much real-world sensor data is needed?
dSPACE conducted a study to investigate the training of neural networks with synthetic sensor data for real-world applications. The goal of the study was to quantify how much real-world data can be reduced when a mixed data set of synthetic and real-world data is used. By modeling the relationship between the number of training examples and recognition performance using a simple power law, it was found that the amount of real-world data required can be reduced by up to 70% without affecting recognition performance. In particular, the training of object recognition networks is improved by enriching the mixed data set with classes that are underrepresented in the real data set. The results show that mixed data sets with real-world data proportions between 5% and 20% reduce the need for real-world data the most, without reducing recognition performance.